sandbox/Antoonvh/kochrefine.c
The Fractal Dimension of the Koch Snowflake without using the adapt_wavelet function.
Another page presents an analysis to find the fractal dimension of the koch snowflake by using the adapt_wavelet function. This page aims to validate a somewhat less involved alternative.
#include "grid/quadtree.h"
#include "navier-stokes/centered.h"
int main(){
int jmax=9;
int n=4;
int nm=((n-1)*pow(4,jmax-1))+1;
double xn[nm],yn[nm];
double xk[nm];
double yk[nm];
xk[0]=0;
xk[1]=1;
xk[2]=0.5;
xk[3]=0;
yk[0]=0;
yk[1]=0;
yk[2]=-sqrt(3.)/2;
yk[3]=0;
fprintf(ferr,"\nyk[n-1]=%g\n\n",yk[n-1]);
for (int j=1;j<jmax;j++){
for (int k=0;k<(n-1);k++){
xn[(k*4)]=xk[(k)];
xn[(k*4)+1]=(2*xk[k]+xk[k+1])/3;
xn[(k*4)+2]=((xk[k]+xk[k+1])/2)+((yk[k]-yk[k+1])*sqrt(3.)/6);
xn[(k*4)+3]=(xk[k]+2*xk[k+1])/3;
yn[(k*4)]=yk[k];
yn[(k*4)+1]=(2*yk[k]+yk[k+1])/3;
yn[(k*4)+2]=((yk[k]+yk[k+1])/2)-((xk[k]-xk[k+1])*sqrt(3.)/6);
yn[(k*4)+3]=(yk[k]+2*yk[k+1])/3;
}
yn[4*(n-1)]=yk[n-1];
xn[4*(n-1)]=xk[n-1];
FILE * fpkoch = fopen("koch.dat","w");
for (int l=0;l<(4*(n-1)+1);l++){
yk[l]=yn[l];
xk[l]=xn[l];
}
if (j==jmax-1){
for (int l=0;l<(4*(n-1)+1);l++){
fprintf(fpkoch,"%g %g\n",xk[l],yk[l]);
}
}
n=(n-1)*4+1;
}
FILE * fpit = fopen("cells.dat","w");
X0=-0.5;
Y0=-1.2;
L0=2.0;
init_grid(32);
scalar c[];
for (int m=0;m<7;m++){
foreach()
c[]=0;
for(int i=0;i<n;i+=64/pow(2,m)){
Point point = locate(xn[i],yn[i]);
c[]+=1.0;
}
int gcc=0;
int gcn=0;
foreach(reduction(+:gcc) reduction(+:gcn)){
gcn++;
if(c[]>0.5)
gcc++;
}
We simply refine the cells that are on the curve. We do not Coarsen cells.
refine((c[]>0.05)&&(level<7+m));
fprintf(fpit,"%d %d %d\n",m,gcc,gcn);
fflush(fpit);
int i=m;
scalar lev[];
foreach(){
lev[]=level;
}
static FILE * fp1 = popen ("ppm2gif --delay 200 > f.gif", "w");
output_ppm (lev, fp1,512,min=4,max=15);
}
}
Results
The adaptive grid that is used to refine the cells in the neighborhood of the curve is visualized below. More red colors represent a higher level of refinement.
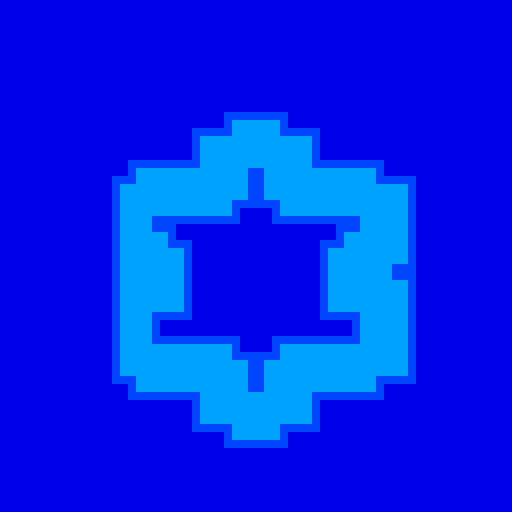
The various stages of refinement
Seems fine?
Now let us check if we can find the fractal dimension of this curve by plotting the number of grid cells against the refinement iteration.
set xr [ -0.5:6.5]
set yr [100:100000]
set logscale y
set xlabel 'refinement iteration'
set ylabel 'Grid cells on the curve'
plot "cells.dat" title "Number of cells on the curve" ,\
(2**(1.26*x))*200 title "2^{1.26x}"
(script)
Apparently the fractal dimension is still 1.26
We check if the total employed number of gridcells scales with the fractal dimension of this “\text{problem}”.
set xr [ -0.5:6.5]
set yr [500:1000000]
set logscale y
set xlabel 'refinement iteration'
set ylabel 'Total number of grid cells'
plot "cells.dat" using 1:3 title "Total number of cells" ,\
(2**(1.26*x))*1000 title "2^{1.26x}"
(script)
Conclusion: Simple refinement of cells on the curve also works well. It might even be more robost. For example when analysing a space-filling curve.